What Is the Distinction Between Data Science and Artificial Intelligence?
Table of Contents
ToggleWhat is Data Science in Hindi
Describe data science.
Today, data science is a dominant field in the IT sector that has dominated practically all industries. It is a vast area that mostly focuses on data systems and processes, and it works with these datasets to extract useful information from them. In this field, data serves as the fuel for gaining practical and insightful knowledge about businesses and spotting emerging market trends.
You now understand the fundamentals of data science. However, I am sure you are curious about the various steps involved in this technology. These steps include data extraction, manipulation, maintenance, and visualisation, which allow you to forecast future events.After learning about Data Science, you may be wondering, “What is the need to study and understand data?”
With data, Facebook is revolutionising social media.
Today, Facebook is the dominant social media platform. With millions of users signed up from all over the world, the platform conducts extensive research using Data Science to extract information about its users’ social interactions. It employs advanced Data Science techniques to comprehend user behaviour and, as a result, improve their product or application. This is just one of many possible applications for this technology. As a result, you can conclude that Data Science is one of the most widely used fields today.
What exactly is artificial intelligence (AI)?
In contrast to Data Science, Artificial Intelligence (AI) is the intelligence that machines can possess. It is inspired by natural human intelligence. You can impose and simulate human intelligence in machines using AI. This technology employs a number of algorithms to aid in autonomous action.
Traditional AI algorithms were given a set of objectives to achieve in order to grow. However, more recent and popular algorithms, such as Machine Learning and Deep Learning, enable you to understand the trends and patterns in the given data and, as a result, determine the data’s purpose.
Learning, reasoning, and self-correction are the three main steps in Artificial Intelligence. Furthermore, AI frameworks can be used for deep neural network computations
To gain a better understanding of this technology, consider a real-world application of AI. Let us now look at the application and implementation of AI in the field of personal security.
AI Applications in Personal Security Systems
Have you recently participated in a large public event or flown? You must have noticed the long lines for security checks if you did. This must have been inconvenient and frustrating for you at times, and with a pandemic like Covid-19 sweeping the globe, it can be frightening. False alarms can be eliminated using Machine Learning, a subset of AI. Furthermore, these machines can detect things that human scanners miss during the screening process in airports, concerts, and stadiums, among other places. This can help to speed up security processes and save a lot of time for security officers as well as passengers and event attendees.
Data Science and Artificial Intelligence (AI
scope and Purpose:
Data Science:
Data science is a multidisciplinary field that primarily deals with extracting insights and knowledge from data. It encompasses various techniques for data collection, cleaning, analysis, and visualization. Data scientists use statistical methods and machine learning to uncover patterns, trends, and correlations in data. The primary goal is to make data-driven decisions and solve real-world problems using data.
Artificial Intelligence (AI):
AI is a broader field that focuses on creating intelligent systems that can perform tasks typically requiring human intelligence, such as understanding natural language, recognizing patterns, making decisions, and solving problems. AI includes various subfields like machine learning, natural language processing, computer vision, and robotics. The primary goal of AI is to develop systems that can simulate human-like intelligence and reasoning.
Methods and Techniques:
Data Science:
Data scientists employ a wide range of techniques, including statistical analysis, data mining, data visualization, and machine learning. Machine learning is a subset of data science and plays a significant role in predictive modeling and pattern recognition.
Artificial Intelligence:
AI relies heavily on machine learning, deep learning (a subset of machine learning), and neural networks to develop models that can learn from data and make predictions or decisions. While AI encompasses other techniques, machine learning is a core component used to build AI models.
Applications:
Data Science:
Data science is used in a wide array of applications, including business analytics, marketing optimization, fraud detection, healthcare analytics, and more. It focuses on extracting insights and generating predictions from data to inform decision-making.
Artificial Intelligence:
AI applications include autonomous vehicles, chatbots, virtual assistants, recommendation systems, image recognition, language translation, and autonomous robotics. AI systems are designed to perform tasks with varying degrees of autonomy and human-like intelligence.
Data vs. Intelligence:
Data Science:
Data science is primarily concerned with working with data, both structured and unstructured, to extract valuable information and insights. It deals with the entire data pipeline from data collection to analysis and visualization.
Artificial Intelligence:
AI focuses on creating systems that can use data to make intelligent decisions or perform tasks that typically require human intelligence. While data is a crucial component of AI, it is just one element in the broader pursuit of achieving machine intelligence.
In summary, data science is a subset of AI, with a specific focus on data analysis and insights extraction, while AI encompasses a broader spectrum of intelligent system development. Both fields are interconnected and often work together, with data science providing the foundation for AI models and AI enabling data-driven decision-making through advanced automation and intelligence.
What Is the Distinction Between Data Science and Artificial Intelligence?
Parameters | Data science | Artificial Intelligence |
Basics | Data Science is a comprehensive process that includes pre-processing analysis, visualisation, and prediction. | Artificial intelligence aids in the implementation of data and machine knowledge. |
Skill | For development and design, you must employ statistical techniques. | You must use algorithms for development and design |
Techniques | Data Science makes use of the Data Analytics technique | Deep Learning and Machine Learning techniques are used in AI. |
Build | By using the concept of data science, we can build complex models about statistics and facts about data. | By using this we emulate cognition and human understanding to a certain level. |
Technique | It uses the technique of data analysis and data analytics. | It uses a lot of machine learning techniques. |
Use | Data science makes use of graphical representation. | Artificial intelligence makes use of algorithms and network node representation. |
Knowledge | ts knowledge was established to find hidden patterns and trends in the data. | its knowledge is all about imparting some autonomy to a data model. |
Examples of Tools | R, Python, etc. are the tools used in data science. | Tensor flow, sci-kit-learn, Kaffee, etc are the tools used in AI. |
models | In Data Science, models are created to generate statistical insights for decision-making. | AI uses Deep Learning and Machine Learning techniques |
observation | It looks for patterns in data to make well-informed decisions | It instils intelligence in machines through the use of data, causing them to respond in the same way that humans do. |
Examples | Process optimization, Customer trends, and financial analysis are some examples. | Robots, Chatbots, online gaming, and voice assistants are some examples |
appllication | Applications of Data Science are dominantly used in Internet search engines, such as Yahoo, Bing, Google, etc. | Applications of Data Science are dominantly used in Internet search engines, such as Yahoo, Bing, Google, etc. |
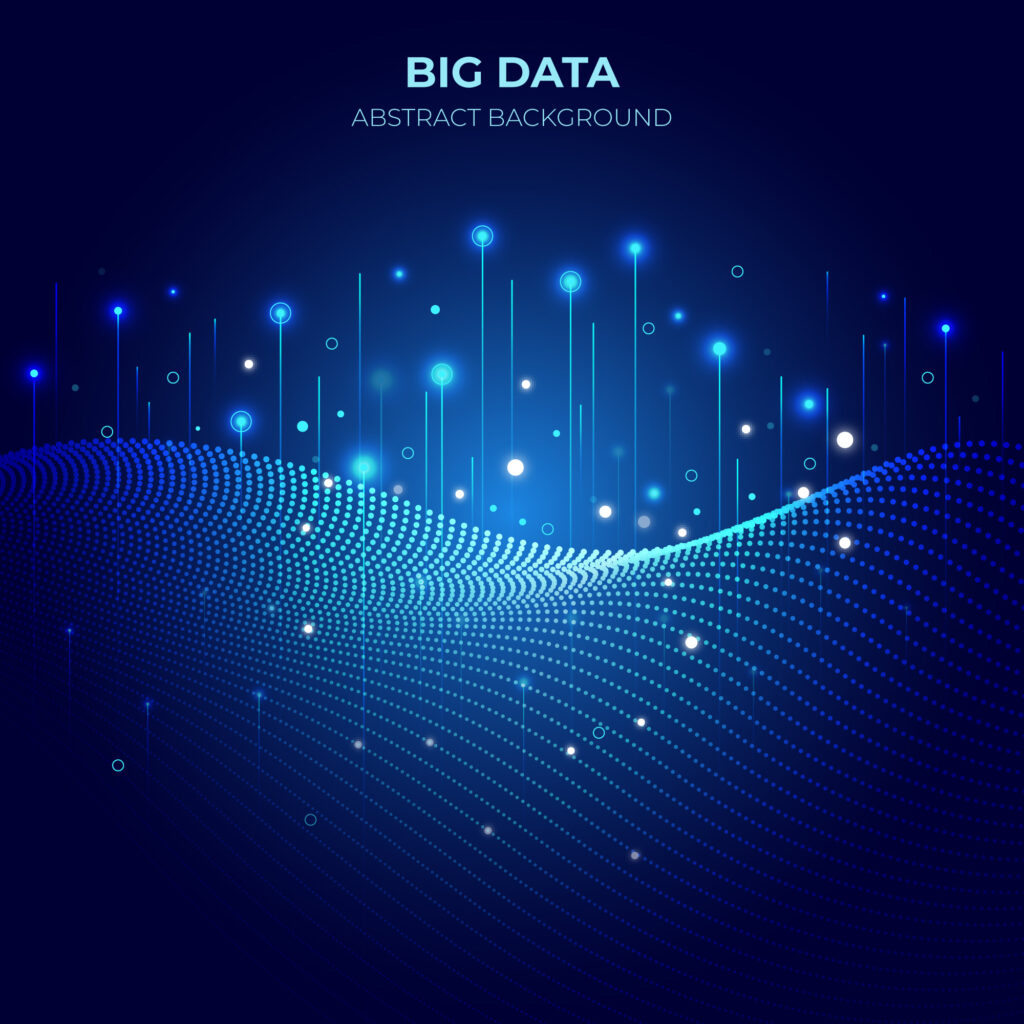
choosing between a career in data science and analytics
1. Take into account your background.
To assist firms make more strategic decisions, data analysts analyse enormous data sets to find trends, build charts, and produce visual presentations. Analysts generally seek undergraduate STEM degrees, and perhaps even graduate degrees in analytics or a similar discipline, to ensure that their education is in line with these objectives. Additionally, they look for candidates with background in science, math, programming, databases, modelling, and predictive analytics.
Data scientists need to have “more of a foundation in computer science,” he adds, adding that they are “far more technical and mathematical [than data analysts].”
It’s crucial to study these educational prerequisites while deciding which job route is best for you. You will probably have the educational and professional background to pursue either path if you have already decided to invest in your career with an advanced degree. On the other hand, since employers are more likely to consider candidates without a master’s degree for these positions, you might be more inclined to stick with a data analytics role if you’re still debating whether returning to school is the right choice for you.
Consider your interests.
Data scientists need to have “more of a foundation in computer science,” he adds, adding that they are “far more technical and mathematical [than data analysts].”
It’s crucial to study these educational prerequisites while deciding which job route is best for you. You will probably have the educational and professional credentials to pursue either option if you have previously decided to invest in your career with an advanced degree. On the other hand, since companies are more likely to consider people without a master’s degree for these positions, you could be more motivated to continue with a data analytics role if you’re still debating whether returning to school is the correct choice for you.
3. Take into account the salary and career path you want.
Earning potential for data analysts is from $83,750 to $142,500. However, because these professionals mostly work with databases, they can raise their wages by learning new programming languages like R and Python.
Data analysts with more than ten years of experience, however, frequently take advantage of their earning potential and move on to other positions, according to PayScale. Following the completion of an advanced degree, two common career changes, according to Blake Angove, director of technology services at IT staffing firm LaSalle Network, include moving into a developer or data scientist position.
Data scientists are regarded as being more senior than data analysts because they frequently possess advanced skills, a doctorate degree, and more experience. As a result, they frequently receive more pay for their services. RHT estimates that the average annual salary for data scientists is between $105,750 and $180,250.
What data profession is best for you?
Given the considerable variances in function responsibilities, educational requirements, and career paths, data analysts and data scientists have job titles that are misleadingly identical.
Regardless of your perspective, Schedlbauer argues that qualified candidates for data-focused occupations are in high demand on the job market right now because of how strongly firms need to make sense of—and profit from—their data.
You can choose the career that is the best fit for you and get started on your path to success once you have taken into account factors like your background, interests, and desired salary.
Data science primarily aims to extract insights and knowledge from data to solve specific problems and make informed decisions. In contrast, artificial intelligence focuses on creating intelligent systems that can mimic human-like reasoning, learning, and decision-making.
Data science involves techniques such as data collection, data cleaning, data visualization, statistical analysis, and machine learning. Machine learning is often used within data science to build predictive models.
Artificial intelligence relies heavily on machine learning, deep learning, neural networks, reinforcement learning, and natural language processing (NLP). These techniques enable AI systems to learn from data and perform intelligent tasks.
Data science has a broader scope and can be applied to various domains, while artificial intelligence often focuses on specific tasks, such as image recognition, speech recognition, and autonomous driving.
In data science, data is the central focus, and the emphasis is on extracting insights and information from data. In artificial intelligence, data is used to train models and enable systems to make intelligent decisions.
Yes, both fields draw from various disciplines. Data science incorporates elements of statistics, computer science, domain expertise, and data engineering. Artificial intelligence is a subfield of computer science and often requires specialized knowledge in machine learning, neural networks, and cognitive science.
Yes, data science is often a component of artificial intelligence. AI systems rely on data and machine learning techniques, which are key elements of data science, to achieve their intelligent behavior.
Data science finds applications in various domains, including finance, healthcare, marketing, and more. It is used for tasks such as customer segmentation, fraud detection, and predictive maintenance.
Artificial intelligence is applied in tasks such as image recognition, natural language processing, autonomous vehicles, recommendation systems, and playing games like chess and Go.