Data Analysis with Power BI 10 Inspiring Project Ideas for Success
Power BI projects emphasize the data analysis and visualization capabilities of Power BI. These businesses may use Power BI to build advanced and appealing dashboards, reports, and visualizations
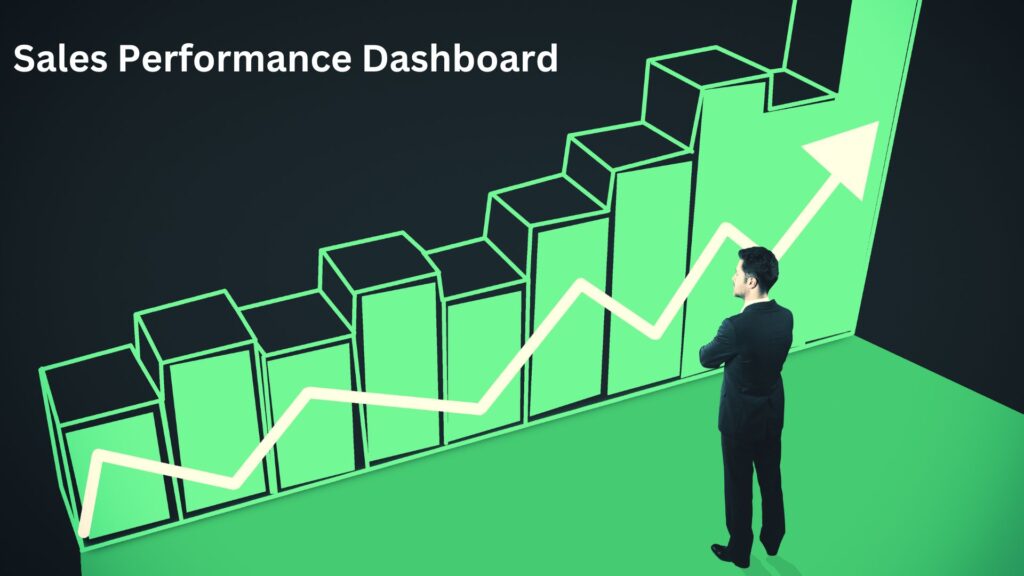
Sales Performance Dashboard
Creating a sales performance dashboard in Power BI can provide valuable insights into your sales data and help track key metrics. Here’s an outline of how you can approach building a sales performance dashboard:
Identify Key Performance Indicators (KPIs): Determine the key metrics that you want to track in your sales performance dashboard. These may include total revenue, sales growth, average deal size, win/loss ratio, customer acquisition cost, and sales by product, region, or salesperson.
Data Integration: Gather the necessary data from your sales systems, such as CRM software or sales databases. Import and integrate the data into Power BI. Ensure the data is clean and properly formatted for analysis.
Design the Dashboard Layout: Plan the layout of your dashboard based on the KPIs identified earlier. Decide on the visualizations you want to include, such as line charts, bar charts, pie charts, and tables. Arrange the visualizations in a logical and intuitive manner for easy interpretation.
Revenue and Sales Metrics: Create visualizations that show revenue trends over time, such as a line chart displaying monthly or quarterly sales figures. Show total revenue, revenue by product category, or revenue by region. Use slicers or filters to allow users to drill down into specific time periods or segments.
Sales Performance by Salesperson: Analyze individual sales performance by creating visualizations that show sales by salesperson, their contribution to overall revenue, and any other relevant metrics. Include a leaderboard or ranking to compare salespeople based on their performance.
Sales Funnel Analysis: Visualize the sales funnel to understand the progression of leads through the sales process. Create a funnel chart to represent the number of leads at each stage, from lead generation to conversion. Analyze conversion rates at each stage to identify bottlenecks and areas for improvement.
Geographic Analysis: If applicable, include maps to visualize sales performance by region or territory. Use choropleth maps or bubble maps to show revenue or sales volume by geographical area. This can help identify high-performing regions and target areas for sales growth.
Performance Against Targets: Compare actual sales performance against targets or quotas. Create visualizations that show progress towards sales targets, either as a bar chart or a gauge chart. Highlight areas where performance is exceeding or falling short of targets.
Drill-Down and Interactivity: Enable users to interact with the dashboard and explore the data in more detail. Add interactivity through slicers, filters, or drill-through actions. Allow users to select specific products, time periods, or salespeople to view detailed information.
Mobile-Friendly Design: Ensure your sales performance dashboard is optimized for mobile devices. Power BI provides options to create responsive designs, allowing users to access and view the dashboard on their smartphones or tablets.
Remember to regularly update and refresh your data in Power BI to keep the dashboard up to date. This will help you monitor sales performance, identify trends, and make data-driven decisions to improve your sales strategies

Customer Segmentation Analysis
Customer Segmentation Analysis is a powerful application of Power BI that helps businesses understand their customer base and target them more effectively. Here’s an overview of a Customer Segmentation Analysis project using Power BI:
Data Collection: Gather customer data from various sources, such as CRM systems, transaction databases, or online platforms. This data may include demographics, purchase history, behavior, interactions, and any other relevant information.
Data Cleaning and Preparation: Cleanse and preprocess the customer data to ensure accuracy and consistency. Handle missing values, standardize formats, and remove duplicates or outliers as needed. Transform the data into a suitable format for analysis.
Identify Segmentation Criteria: Determine the criteria or variables based on which you want to segment your customers. This could be demographics, purchase behavior, preferences, geographic location, or any other relevant factors that differentiate your customer base.
Segmentation Model Development: Utilize Power BI’s data modeling capabilities to develop a segmentation model. Apply appropriate algorithms, such as clustering techniques (e.g., k-means, hierarchical clustering) or decision trees, to segment the customers based on the identified criteria.
Visualize Customer Segments: Create visualizations in Power BI that represent the different customer segments. Use charts, graphs, or tables to display the characteristics, sizes, and distributions of each segment. Highlight the key differentiating factors for each segment.
Segment Profiling: Analyze the characteristics and behaviors of each customer segment. Create visualizations that showcase the segment-specific metrics, such as average purchase value, frequency, customer lifetime value, or customer satisfaction scores. Compare and contrast the segments to identify unique patterns and opportunities.
Targeted Marketing Strategies: Based on the customer segments’ profiles, develop targeted marketing strategies and campaigns. Utilize Power BI’s capabilities to track the effectiveness of these strategies over time and assess their impact on customer behavior and outcomes.
Interactive Exploration: Enable interactive exploration of the customer segments within the Power BI dashboard. Implement slicers or filters that allow users to drill down into specific segments or compare multiple segments. This interactivity helps in gaining deeper insights and understanding of customer behavior.
Reporting and Sharing: Design comprehensive reports in Power BI that summarize the findings from the customer segmentation analysis. Share these reports with key stakeholders, such as marketing teams, sales teams, or senior management, to drive data-informed decision-making and align business strategies.
Continuous Iteration and Improvement: Customer segmentation is an ongoing process. Continuously monitor and evaluate the effectiveness of your customer segments and update them as needed. Incorporate feedback and new data to refine the segmentation model and optimize marketing efforts.
By conducting a Customer Segmentation Analysis project in Power BI, businesses can gain a deeper understanding of their customer base, tailor their marketing strategies, and improve customer engagement and satisfaction.
Customer Churn Analysis

Analysing customer behaviour and determining the causes of customer attrition or churn are part of Power BI’s customer churn analysis. Power BI is an effective tool for data visualization that can be used to build interactive reports and dashboards that reveal patterns of client attrition. Here is a general procedure for running a Power BI customer churn analysis:
Data Preparation: Start by gathering relevant data sources such as customer information, transaction history, customer interactions, and churn status. Clean and organize the data, ensuring it is in a format suitable for analysis.
Data Modeling: Import the prepared data into Power BI and create a data model. Identify key fields such as customer ID, churn status, transaction dates, product or service usage, and any other relevant variables.
Measure Definition: Define measures that will help you analyze churn. These may include churn rate, customer lifetime value, average tenure, or any other metrics specific to your business.
Visualization Creation: Create visualizations to explore churn patterns. Common visualizations may include churn trend over time, churn by customer segment, churn by product/service, or customer retention rate. Use Power BI’s drag-and-drop interface to create these visualizations.
Cohort Analysis: Perform cohort analysis to understand how customer cohorts behave over time. This can help identify differences in churn rates between different groups of customers, such as new customers vs. long-term customers.
Predictive Modeling: Utilize machine learning algorithms available in Power BI to build predictive models for churn. These models can help identify customers at risk of churning based on historical data and predictive variables.
Interactive Dashboards: Create interactive dashboards that allow users to drill down into specific customer segments or time periods. Include filters, slicers, and other interactive elements to enable deeper exploration of the data.
Report Sharing: Share the churn analysis report or dashboard with stakeholders within your organization. Power BI allows you to publish reports to the Power BI service, where users can access and interact with the analysis.
Energy Trade Analysis
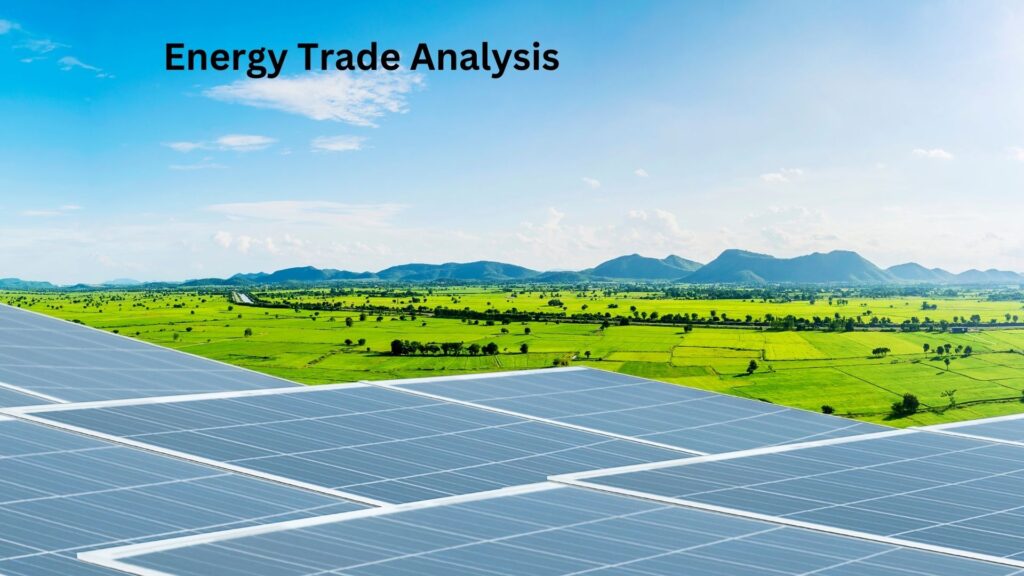
This study examines a wide range of problem of global energy production and trade. This project looks at a wide range of topics, including the growth of wind energy, comparing the economy of other nations based on their energy usage, and much more.
Use the dataset of global energy statistics for this project idea. As a result of this effort, Power BI dashboards will provide overall energy statistics, including primary and secondary energy production, trade, and consumption, new and renewable energy sources, as well as conventional and unusual energy sources. In this project, a number of scenarios can be handled using Power BI data visualisation capabilities, as shown below:
Use bar charts, cards, flow diagrams, etc. for the overview/summary page; for the pages
Social media Analytics
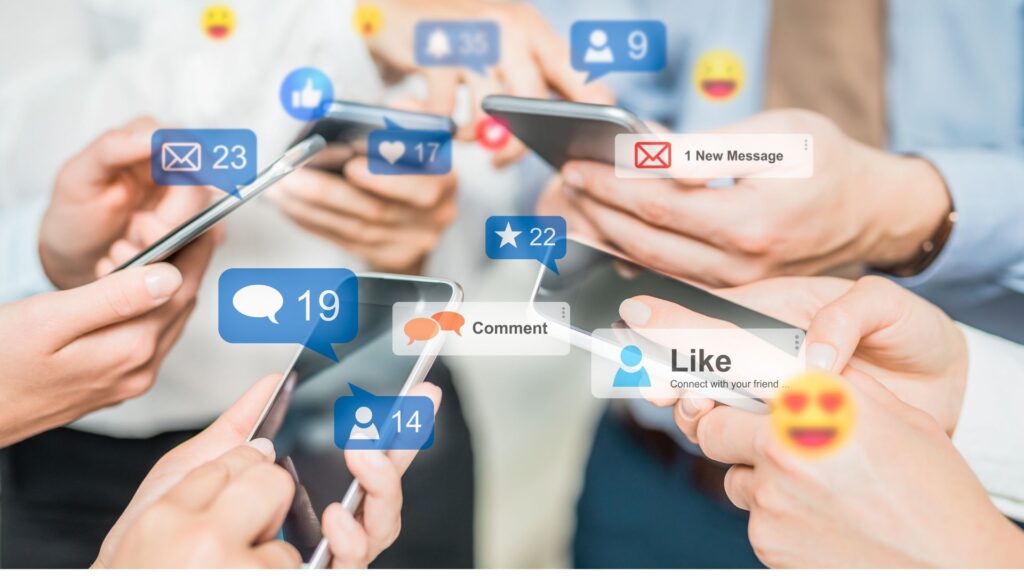
In today, scenario Social media analytics in Power BI involves analyzing data from social media platforms to gain insights into audience engagement, sentiment analysis, campaign performance, and other relevant metrics. Power BI can help you create interactive reports and visualizations to understand social media data and make data-driven decisions.
By connecting Power BI to social media platforms such as Twitter, Facebook, LinkedIn, or Instagram, you can retrieve data such as posts, comments, likes, shares, follower counts, and other relevant metrics. Once the data is imported into Power BI, you can perform data preparation tasks, define measures, and create visualizations to analyze the social media data.
For audience engagement analysis, you can track engagement metrics over time, identify popular posts or topics, and measure the reach and impact of your social media activities. Sentiment analysis techniques can be applied to understand the sentiment (positive, negative, or neutral) of social media posts or comments, providing insights into public opinion or customer sentiment towards your brand or products.
Campaign performance analysis is another important aspect of social media analytics. By monitoring campaign-specific metrics such as reach, impressions, click-through rates, or conversions, you can assess the effectiveness of your social media campaigns and make data-driven decisions to optimize your marketing strategies
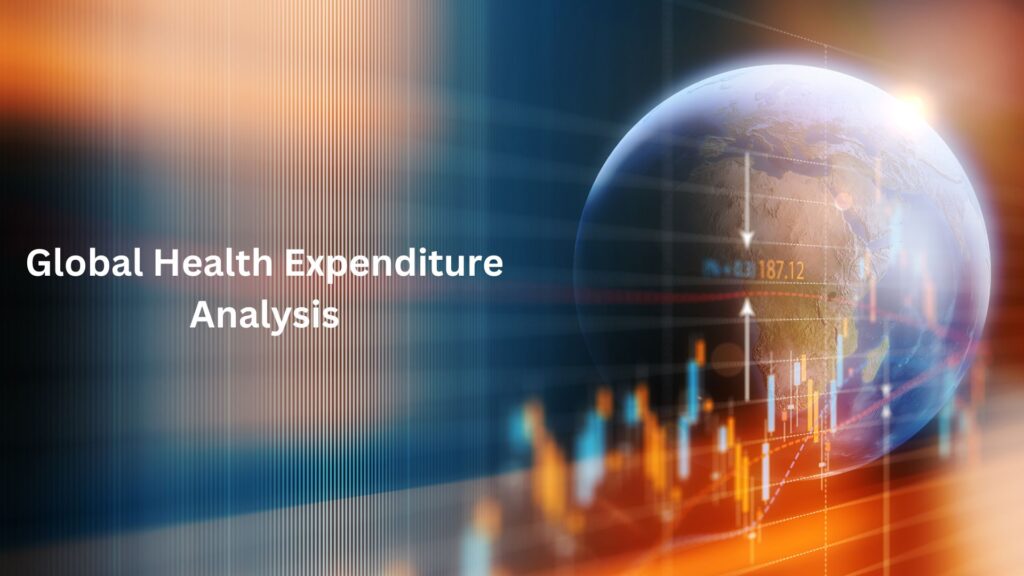
Global Health Expenditure Analysis
Comparative information on health spending for 192 nations over the past 20 years is provided through a worldwide study of health expenditure. In this project, Power BI clustering analysis is implemented using PyCaret, a Python machine learning toolkit. Gathering data items with related properties is known as clustering. These classifications support data analysis, pattern detection, and dataset examination, while data clustering supports the discovery of underlying data structures.
You can use the most recent health spending dataset from the WHO Global Health Expenditure Database for this project. The K-Means technique is another option for your clustering study. You may view the cluster labels in Power BI Dashboard in order to obtain insights after loading the dataset into Power BI Desktop and training your clustering model there. For the summary page dashboard, you may select visualisation chart kinds including bar charts and filled maps, while point maps could be used for the comprehensive visualisation.
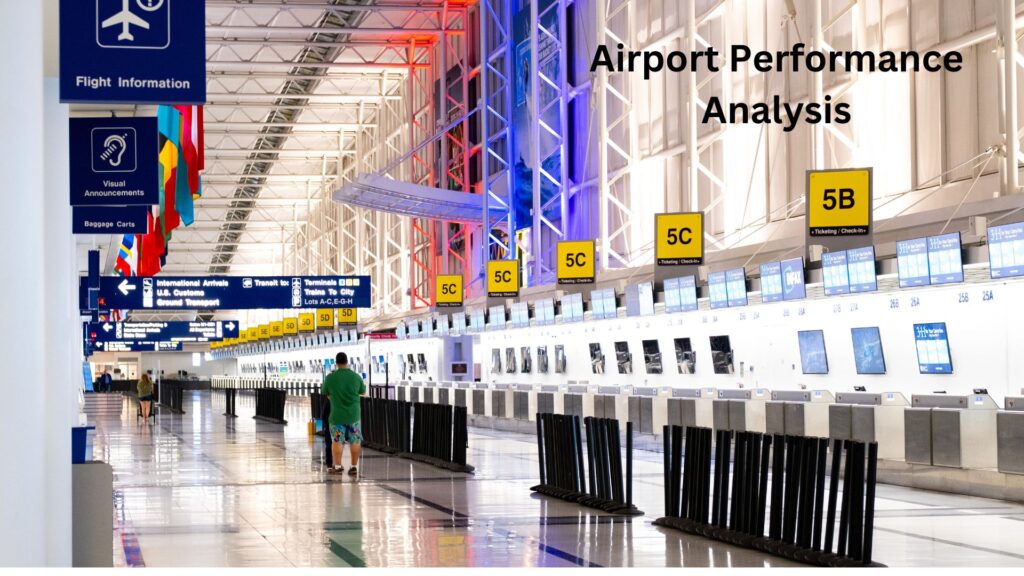
Airport Performance Analysis
Passenger numbers in the aviation sector increased dramatically on a global scale. Over 5,000 airplanes are currently transporting people and freight all over the world. However, keeping up with these flights demands a lot of preparation and rapid judgment. In this situation, Power BI is useful.
Airport data will be analysed as part of the Airport Authority Data Analysis initiative to give a clear picture. The number of flights (incoming and outgoing), the number of delays (arrivals and departures), passenger comments, and ground processing times are all displayed on the summary page of Power BI dashboards. This kind of research might help airport management authorities make quick choices in the event of flight adjustments, crises, or delays. This project concept can make use of the dataset for airline delays and cancellations. Power BI data visualisation options, as listed below, may be utilised for various scenarios in this project.
For flight analysis, there are cards, bar charts, tornado charts, tree maps, flow maps, etc.
For the passenger input, there are cards, bar charts, column charts, etc.
Supply Chain Optimization
Use Power BI to analyze supply chain data, monitoring inventory levels, lead times, supplier performance, and distribution efficiency to optimize your supply chain processes.
Supply chain optimization is a complex but rewarding project that involves improving the efficiency and effectiveness of the processes involved in the production and distribution of goods. Here’s how you can approach this project using Power BI:
1. Data Collection and Integration:
Gather data from various sources within your supply chain, including suppliers, warehouses, transportation, and production facilities.
Integrate this data into a centralized database or data warehouse for analysis.
2. Key Performance Indicators (KPIs) Selection:
Identify the key metrics that are crucial for evaluating supply chain performance, such as inventory turnover, lead time, order fulfillment rate, and on-time delivery.
3. Data Analysis and Visualization:
Design a Power BI dashboard to visualize your supply chain data. Use visuals like line charts, bar charts, heat maps, and geographical maps to represent data trends and patterns.
Create visuals that show the flow of goods from suppliers to customers, highlighting potential bottlenecks or areas for improvement.
4. Demand Forecasting:
Implement forecasting models to predict future demand for your products. This can help optimize inventory levels and production schedules.
5. Inventory Management:
Analyze inventory levels and turnover rates. Visualize the stock levels at different stages of the supply chain, from suppliers to warehouses to customers.
6. Supplier Performance Analysis:
Evaluate the performance of your suppliers based on factors such as lead times, quality, and reliability.
Use Power BI to create scorecards or dashboards that compare different suppliers’ performance metrics.
7. Production Efficiency:
Analyze production processes and identify areas for optimization. Track production cycle times, downtime, and utilization rates.
Visualize production efficiency metrics and identify potential causes of production delays.
8. Transportation and Distribution Optimization:
Visualize transportation routes and distribution networks using geographical maps in Power BI.
Analyze transportation costs, delivery times, and route efficiency. Identify opportunities to optimize routes and reduce transportation expenses.
9. Cost Analysis:
Create visuals that display costs associated with various stages of the supply chain, such as procurement, production, transportation, and warehousing.
Compare these costs to revenue and identify cost-saving opportunities.
10. Scenario Analysis and What-If Scenarios:
Use Power BI to create scenarios that explore the impact of changes in variables like demand, lead times, or production capacity on the overall supply chain performance.
Run “what-if” scenarios to assess the potential outcomes of different decisions.
11. Collaboration and Insights Sharing:
Share your Power BI dashboard with relevant stakeholders across the supply chain, such as suppliers, production managers, and logistics teams. This facilitates collaboration and informed decision-making.
Remember that supply chain optimization is an ongoing effort, and your Power BI dashboard should be regularly updated to reflect the most current data and insights. The goal is to identify areas for improvement and make data-driven decisions that lead to a more efficient and responsive supply chain.
E-commerce Analytics:
Develop a dashboard for an e-commerce business to visualize product sales, cart abandonment rates, conversion rates, and customer demographics. Insights can drive marketing and product strategies.
E-commerce analytics is a critical process that empowers online retailers to uncover valuable insights within the vast sea of data generated by their operations. By collecting and integrating data from diverse sources such as sales databases, website analytics tools, and customer feedback, businesses gain a holistic view of their performance. This data-driven approach enables comprehensive sales analysis, shedding light on revenue trends, customer behaviors, and product popularity. Moreover, understanding customer behavior through segmentation based on demographics, location, and purchasing habits allows for personalized marketing strategies and improved customer experiences.
Through e-commerce analytics, enterprises can optimize their conversion rates by identifying bottlenecks in the purchasing journey and refining website layouts and checkout processes. Web traffic analysis provides granular insights into user behavior, enabling businesses to enhance their online platforms. Additionally, the segmentation of customers aids in inventory management by facilitating the optimization of stock levels, reducing stockouts, and mitigating excess inventory.
By evaluating customer feedback and sentiment analysis, companies can gauge customer satisfaction levels and tailor their offerings accordingly. Marketing campaign analysis uncovers the efficacy of various strategies, while geographic analysis aids in tailoring efforts to specific regions. E-commerce analytics also encompasses cohort analysis, enabling businesses to understand customer retention and lifetime value. Moreover, predictive analytics, integrated within analytics dashboards, empowers forecasting of sales, demand, and potential churn.
Creating interactive dashboards using tools like Power BI enables users to explore multidimensional data easily, fostering informed decision-making. Regularly updating and sharing these insights across teams ensures alignment and maximizes the impact of data-driven decisions. In the dynamic landscape of e-commerce, analytics stands as a guiding light, steering businesses toward growth, optimization, and improved customer satisfaction.