Top 10 Retail Data Science Use Cases [Includes Case Studies]
Table of Contents
ToggleRetail Case Study Data Analytics
A retail case study in data analytics is an analysis of retail data to understand customer behavior, identify patterns, and draw conclusions about how to improve retail performance. This type of study can be conducted at the individual or micro level (e.g. an individual customer’s shopping habits) or at a larger macro level (e.g. patterns in customer spending across a geographic area).
At the individual level, data analytics can be used to identify customer segments and analyze their preferences, buying habits, and loyalty. This can be used to inform decision-making about product selection and pricing, customer acquisition and retention, and marketing campaigns.
At the macro level, data analytics can be used to identify customer trends and patterns of behavior. This can help retailers understand the impact of changes in the market and make informed decisions about how to best serve their customers. For example, a retailer may analyze data about customer spending during economic downturns to determine how to adjust their strategy to best serve their customers during periods of economic instability.
Data analytics can also be used to optimize inventory levels, identify opportunities for cost savings, and determine which marketing campaigns are most effective. By understanding customer behavior, retailers can make informed decisions about their product selection, pricing, and promotions.
Overall, data analytics can provide valuable insights into customer behavior that can help retailers make better decisions about how to best serve their customers.
Understanding client preferences is crucial for retailers to succeed in the retail sector. their preferences, making sales predictions, and controlling overall costs.
Today, it has become incredibly challenging to offer your items to millions of people throughout the world and comprehend their mentalities. Retailers began utilising data science for this reason, which is where data science enters the picture.
Data Science has a significant impact on the retail industry today in a variety of ways. Data Science provides retailers with countless opportunities. Matching the rising expectations of consumers is the primary reason the retail sector uses data science.
The retail sector produces a lot of data about its customers. Data science aids in drawing conclusions about the consumers and market trends from this data. The industries will be able to make some significant data-driven decisions at the appropriate moment thanks to the effective utilisation of this information. By examining the data, retailers may create a variety of customer-influencing methods.
We will examine the top data science use cases in the retail industry in this essay on data science in retail.
Recommendation Systems
The customer’s prior history serves as the foundation for the recommendation system. While customers are browsing the internet, it enables shops to comprehend their interests and provide pertinent recommendations.
In general, recommendation engines either utilise collaborative filtering or content-based filtering. The clients’ prior decisions and favourite items are taken into account during this procedure. The recommendation engines use a variety of algorithms based on this data. Then it comes up with some comparable ideas and presents the users with various products and services in accordance.
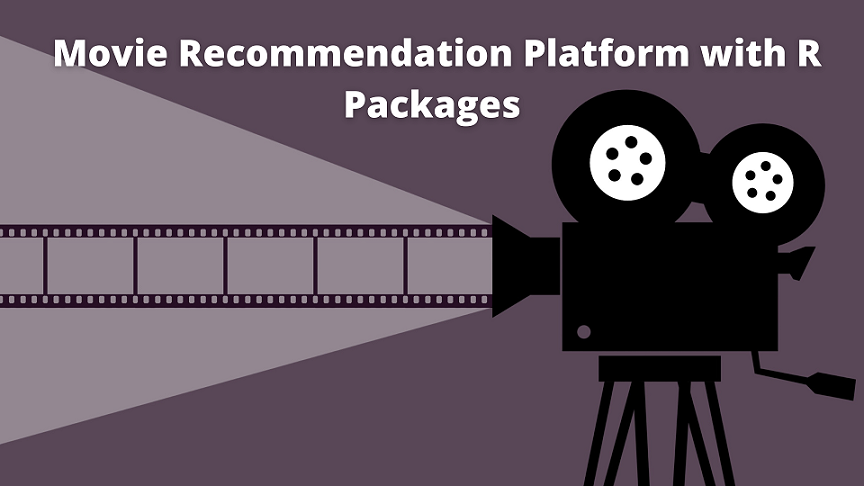
Personalized Marketing
For years, targeted marketing has been profitable for merchants. The procedure entails gathering a sizable amount of data from the transactions of millions of consumers and then analysing it to forecast the customers’ future decisions or choices. This study is based on consumer preferences, complaints, and reviews of various items.
It will assist merchants in making crucial judgments about pricing strategies and giving customers individualised advice.
The data insights obtained assist the industries in enhancing their growth plans, marketing strategies, and sales.
Price Optimization
PWC (PriceWaterhouseCoopers) estimates that 60% of all consumers feel that a product’s price is the primary factor in their decision to buy it.
Data science has developed fresh methods for resolving this issue. The many pricing optimization approaches assist the store in determining the right prices for their goods.
Tools used for pricing optimization include mystery shopping, location information, consumer segmentation, etc.
The pricing optimization model’s many algorithms carry out a real-time study of the consumer’s reaction to prices, discounts, sales during holidays, marketing campaigns, etc.
For instance, Walmart, one of the top worldwide multinational corporations, has created its own data analytics hub, known as Data Cafe. More than 40 petabytes of client data that aids in identifying market trends have become a nuisance to the Data Cafe. The Walmart grocery team received this sophisticated study, which revealed that a certain item’s sale has abruptly decreased due to its inappropriate pricing.
So that the necessary action may be done if there is a rapid drop in a product’s sales, this algorithm warns them.
Inventory Control
The goal of merchants is to satisfy client demands whenever they arise.
The goal of inventory management is to make sure that goods are always available for purchase. Retailers may utilise data science to forecast demand and keep a safety buffer to handle fluctuating demand.
The ability to predict demand will help businesses have more inventory on hand so they can serve clients during emergencies.
To create models, a variety of cutting-edge machine learning methods are used. They are able to identify distinct patterns and connections among the various supply chain components. These algorithms assist the shops in maintaining product inventory in accordance with anticipated sales patterns.
Putting Augmented Reality to use
The retail sector is gradually embracing the concept of augmented reality as a way to let buyers sample a product without really purchasing it..
Users may scan the products at IKEA, one of the top Swedish retailers of furniture and home goods. Customers may scan the goods they want to purchase and digitally place them in their homes to see how they originally appeared. Through the use of picture recognition technology, this will enable people to feel the merchandise.
Before buying the product, customers may have any questions they have answered on the colour, size, etc. This makes it easier for buyers to decide what they want and buy it with confidence. Therefore, the businesses must deal with lower returns and declining sales.
Address of New Stores
Data science seems to be a useful technique for determining the best site to open a new business. Making such selections necessitates the examination of a significant quantity of data, including internet consumer data, local market trends, the locations of other neighbouring businesses, etc.
All of these elements are taken into account by the algorithm when it analyses. The data are then evaluated in a way that aids businesses in making choices about where to locate a new shop.
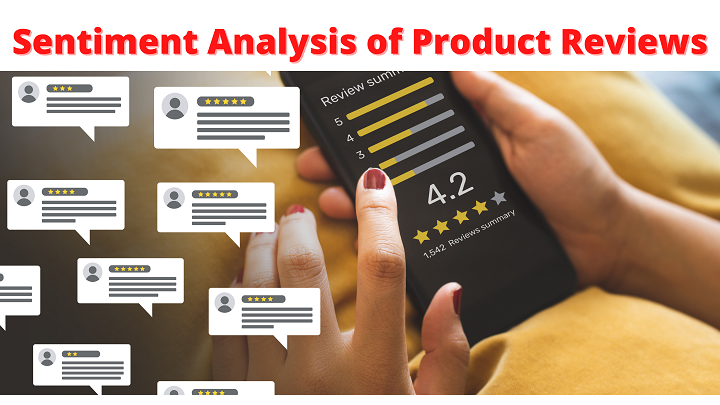
Analysis of customer sentiment
Customer sentiment research is not just important for the retail sector; it also has a big impact on a lot of other sectors. It has become significantly easier because to the application of Data Science in retail.
We analyse customer sentiment using a variety of sophisticated machine learning methods. The algorithm uses client information gathered from online service reviews and social media channels. that they can comprehend the customer’s perspective on the merchandise.
Language processing is used in this study to pinpoint the words that indicate the customer’s attitude, whether it be good or negative. The result reflects the total sentiments of the text after analysis of all the customer feelings that were discovered.
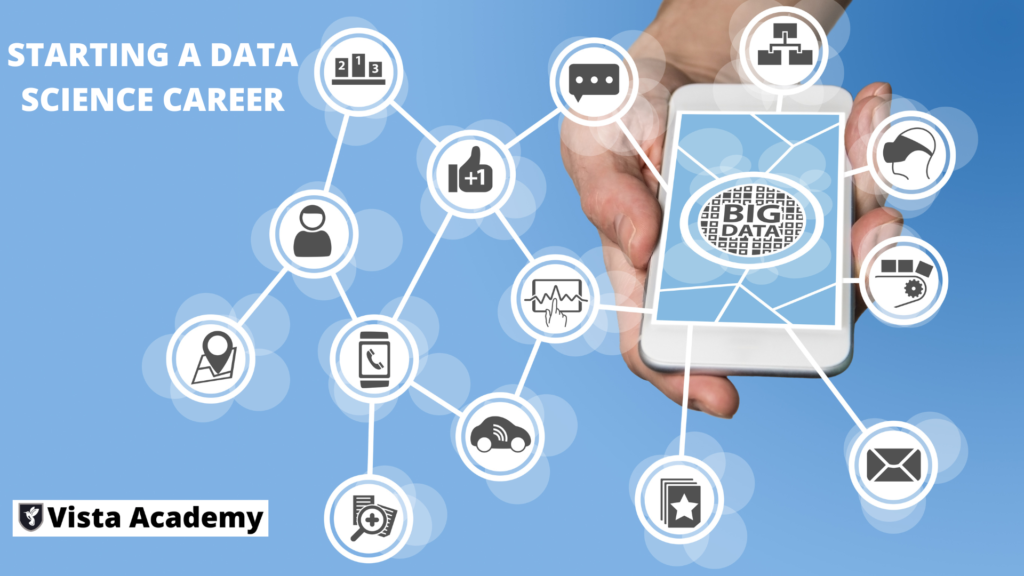
Social Media Usage
Social media is much more than simply a way to stay in touch with pals. It gives businesses a tonne of customer data that aids in their comprehension of consumer trends, behaviours, and buying patterns.
However, you should not break the consumers’ privacy policies when retrieving this data.
For instance, Nordstrom is a well-known fashion shop in the US. It searches via numerous social media sites, like Facebook, Twitter, Instagram, and others, to learn about the most well-liked items and provide them at its retail locations.
NLP (Natural Language Processing) is used to collect the data, and various machine learning techniques are used to extract these important insights from it.
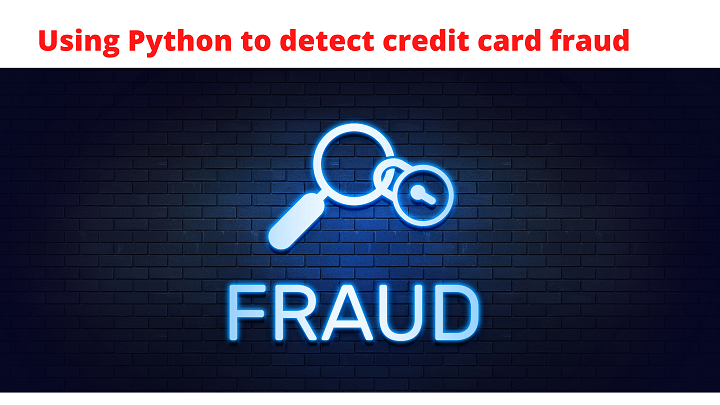
Detecting fraud
Getting the trust of the consumer is one of the key elements impacting the performance of the sector. However, certain fraudulent acts might permanently damage the consumers’ valued confidence, which would be a big loss for the sector.
Merchandising
The reputation of the business is protected through data science in retail. For merchants, detecting fraud is a difficult issue.
Companies are increasingly using a variety of machine learning techniques and deep neural networks after suffering significant financial losses. This makes it possible for them to constantly monitor all actions and stop any fraudulent ones. These algorithms assist in both the detection of fraud and the forecasting of subsequent fraud.
EXAMPLE OF WALMART RETAIL CASE STUDY
The Walmart case study on retail data analytics is an intriguing example. With more than 11,000 shops in 27 countries, Walmart is one of the biggest retail companies in the world. Walmart wanted to eliminate waste and optimise its supply chain by correctly forecasting consumer demand.
Walmart used a data analytics tool to do this by examining sales data at the point of sale, inventory data from the stores, and historical sales data. The system employed machine learning algorithms to forecast store-level demand and adjust the supply chain as necessary. The answer also assisted Walmart in determining which items were overstocked and which were likely to run out of stock.
This implementation had important effects. Walmart was able to cut $2 billion from their inventory, which increased their profit margin by 10%. In addition, Walmart was able to cut food waste by 50 million pounds annually, or 40,000 truckloads of food, thanks to the solution.
As a result, Walmart’s case study provides a fantastic illustration of how retail data analytics can assist merchants in streamlining their supply chains, cutting waste, and boosting their profit margins. Retailers may make data-driven choices and get a competitive edge in the market by utilising the power of data analytics.
Target retail chain data science in retail example
Target, a well-known retail business in the United States, produced another intriguing retail data analytics case study. By making tailored recommendations to each consumer, Target hoped to enhance the customer
Target used a data analytics tool to do this, analysing consumer information including purchase and browsing history as well as demographic information. The system made customised product suggestions based on machine learning algorithms that predicted the things a consumer is likely to buy.
experience.
This implementation had important effects. Target was able to enhance customer satisfaction while increasing sales by 10%. Higher client loyalty and repeat business were other results of the personalised suggestions.
Starbuck Retail Analytics case studies
The case study of Starbucks is a further illustration of retail data analytics. Starbucks sought to enhance customer experience and optimise shop placements. They used a data analytics system to do this by examining consumer, store performance, and location data.
The solution predicted the ideal retail locations and store layout using machine learning methods. Based on their location and past purchases, the service also gave clients personalised recommendations.
This implementation had important effects. Starbucks was able to boost revenues by 15% while also enhancing the customer experience. Higher client loyalty and repeat business were other results of the personalised suggestions.
In conclusion, these case studies show how retail data analytics can benefit merchants by enhancing consumer satisfaction, boosting sales, and gaining a competitive edge. Retailers can make data-driven choices and give their consumers a better shopping experience by utilising the power of data analytics.